The Kore.ai NLP engine uses Machine Learning, Fundamental Meaning, and Knowledge Graph (if any) models to match intents. All the three Kore.ai engines finally deliver their findings to the Kore.ai Ranking and Resolver component as either exact matches or probable matches. Ranking and Resolver determines the final winner of the entire NLP computation.
The NLP engine uses a hybrid approach using Machine Learning, Fundamental Meaning, and Knowledge Graph (if the bot has one) models to score the matching intents on relevance. The model classifies user utterances as either being Possible Matches or Definitive Matches.
Definitive Matches get high confidence scores and are assumed to be perfect matches for the user utterance. In published bots, if user input matches with a single Definitive Match, the bot directly executes the task. If the utterances match with multiple Definitive Matches, they are sent as options for the end user to choose one.
On the other hand, Possible Matches are intents that score reasonably well against the user input but do not inspire enough confidence to be termed as exact matches. Internally the system further classifies possible matches into good and unsure matches based on their scores. If the end user utterances were generating possible matches in a published bot, the bot sends these matches as “Did you mean?” suggestions for the end user.
Based on the ranking and resolver, the winning intent between the engines is ascertained. If the platform finds ambiguity, then an ambiguity dialog is initiated. The platform initiates one of these two system dialogs when it cannot ascertain a single winning intent for a user utterance :
- Disambiguation Dialog: Initiated when there are more than one Definitive matches returned across engines. In this scenario, the bot asks the user to choose a Definitive match to execute. You can customize the message shown to the user from the NLP Standard Responses.
- Did You Mean Dialog: Initiated if the Ranking and Resolver returns more than one winner or the only winning intent is an FAQ whose KG engine score is between lower and upper thresholds. This dialog lets the user know that the bot found a match to an intent that it is not entirely sure about and would like the user to select to proceed further. In this scenario, the developer should identify these utterances and train the bot further. You can customize the message shown to the user from the NLP Standard Responses.
NLP Detection
The Natural Language Analysis will result in the following scenarios:
- NLP Analysis identifying a Definitive match with FM or ML or KG engines
- NLP Analysis with Multiple engines returning probable match and selecting a single match
- NLP Analysis with Multiple engines returning probable match and resolver returning back multiple results
- NLP Analysis with No match
Each of the above cases is discussed in this section.
To understand NLP detection, let us use the example of a Bank bot with the following details:
Scenario 1 – NLP Analysis with FM identifying a Definitive match
- The Fundamental meaning(FM) model identified the utterance as a Definitive match.
- The Machine Learning (ML) model also identified it as a Possible match.
- The score returned for the task identified is 6 times more than other intent scores. Also, all the words in the intent name are present in the user utterance. Thus the FM model termed it a Definitive match.
- The ML model matches the Find ATM intent as a Probable match.
Scenario 3 – NLP Analysis with KG identifying a Definitive match
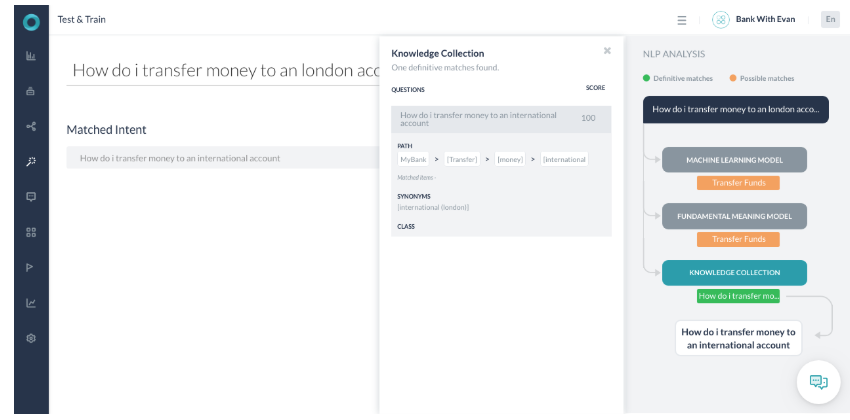
- The user utterance is “How do I make transfer money to a London account?”
- The user utterance contains all the terms required to match this Knowledge task path Transfer, Money, International.
- The term international is identified as a synonym of London that the user used in the utterance.
- As 100% path term matched the path was qualified. As part of confidence scoring, the terms in the user query are similar to that of the actual Knowledge task question. Thus, it returns a score of 100.
- As the score returned is above 100, the intent is marked as a Definitive match and selected.
- FM engine found it a Probable match as the key term Transfer is present in the user utterance
- ML engine found the utterance as a Probable match as the utterance did not fully match any trained utterance.
Scenario 4 – NLP Analysis with Multiple engines returning probable match and selecting a single match
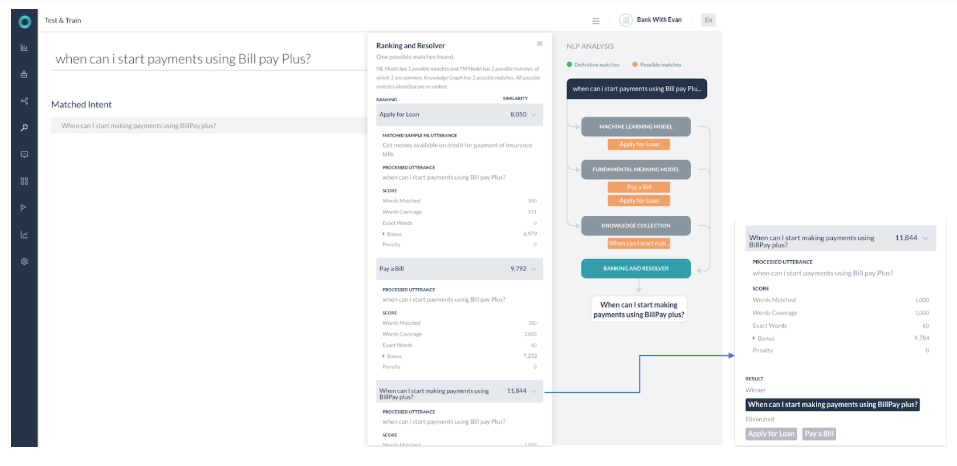
- All the 3 engines returned possible match and no definitive match
- ML Model has 1 possible matches and FM Model has 2 possible matches, of which 1 is common. Knowledge Graph has 1 possible match. All possible matches identified are re-ranked in the Ranking and Resolver.
- The Ranking and Resolver component returned the highest score for the single match (Task name – “ When can I start making payments using BillPay plus? ”) from Knowledge graph engine. The scores for other probable match come out to be lower than 2 percentile of the top score and are thus ignored. The winner, in this case, is the ‘KG’ returned query and is presented to the user.
- Though most of the keywords in the user utterance map to the keywords in the KG query, still this is not a definitive match because
- The number of path term matched are not 100%
- The KG engine returned the score with 64.72% probability. Had we used the word ‘Billpay’ instead of ‘bill pay’ the score would have been 87.71%. (still not a 100% match)
- Now as the score is between the 60%-80% threshold the Query is presented as part of the ‘Did-you-mean’ dialog and not as a complete winner. If the score was above 80% the platform would have given out the response without re-confirming with the ‘Did-you-mean’ dialog.
Scenario 5 – NLP Analysis with Multiple engines returning probable match and resolver returning back multiple results
- All the engines detected probable matches
- KG returned with 2 possible paths
- Ranking and resolver found the 2 queries with a score less than 2% apart.
- Both the Knowledge tasks are selected and presented to the user as ‘Did-you-mean’
- Both the paths were selected as terms in both matched and the score for both the paths is more than 60%